Uncovering the Relationship Dynamics of Data Mining Applications
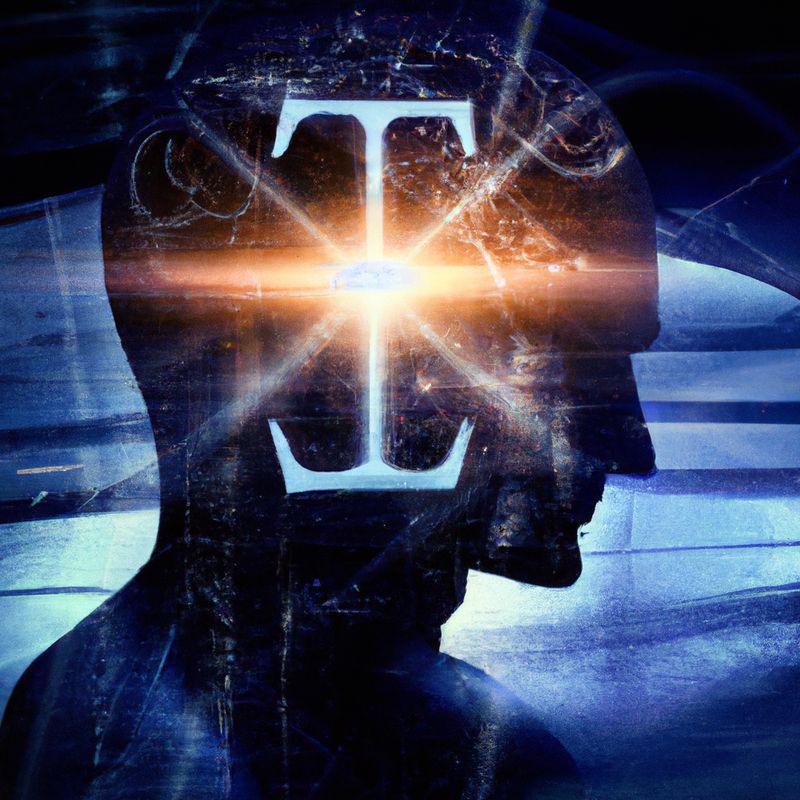
Data mining applications have become increasingly popular in recent years as businesses and organizations strive to make the most of their data. In order to understand the relationship dynamics of data mining applications, it is important to look at the various types of data mining, the techniques used, and the potential implications of using data mining in various contexts. This article will explore the relationship dynamics of data mining applications and how they can be used to gain insights into customer behavior, market trends, and other valuable data.
What is Data Mining?
Data mining is a process of extracting useful information from large datasets. It involves the use of various techniques and algorithms to identify patterns and correlations in data. Data mining can be used to uncover hidden relationships and trends in data that may not be apparent from the raw data. This type of analysis is often used in business intelligence, marketing, and other areas where data can be used to gain insights into customer behavior, market trends, and other valuable data.
Types of Data Mining
There are several types of data mining that can be used to uncover hidden relationships and trends in data. The most common types of data mining are association rule mining, cluster analysis, decision tree analysis, and neural networks. Each of these techniques has its own strengths and weaknesses and can be used to uncover different types of relationships and trends in data.
Association Rule Mining
Association rule mining is a type of data mining that is used to uncover relationships between items in a dataset. This type of data mining looks for patterns in the data that indicate a correlation between the items in the dataset. For example, if a dataset contains information about customers and their purchases, association rule mining can be used to uncover relationships between items that customers tend to purchase together. This type of analysis can be used to uncover relationships between products, customers, and other factors that can be used in marketing and other areas.
Cluster Analysis
Cluster analysis is a type of data mining that is used to identify groups of similar items in a dataset. This type of analysis can be used to identify clusters of customers, products, or other factors that have similar characteristics. For example, cluster analysis can be used to identify groups of customers who have similar purchase patterns or who are likely to respond to a certain type of marketing campaign. This type of analysis can be used to segment customers into different groups for targeted marketing or other purposes.
Decision Tree Analysis
Decision tree analysis is a type of data mining that is used to uncover relationships between different variables in a dataset. This type of analysis is often used to uncover relationships between different factors that may influence a certain outcome. For example, decision tree analysis can be used to identify relationships between customer demographics, purchase history, and other factors that may influence a customer's likelihood of making a purchase. This type of analysis can be used to create predictive models that can be used to make more accurate predictions about customer behavior.
Neural Networks
Neural networks are a type of data mining that uses artificial intelligence to uncover relationships between different variables in a dataset. Neural networks are often used to uncover hidden patterns and correlations in data that may not be apparent from the raw data. This type of analysis can be used to uncover relationships between different factors that may influence a certain outcome. For example, neural networks can be used to identify relationships between customer demographics, purchase history, and other factors that may influence a customer's likelihood of making a purchase.
Implications of Data Mining
Data mining can be used to uncover hidden relationships and trends in data that can be used to gain insights into customer behavior, market trends, and other valuable data. However, it is important to consider the implications of using data mining in various contexts. For example, data mining can be used to identify patterns in customer data that can be used to target customers with specific products or services. This type of analysis can be used to create predictive models that can be used to make more accurate predictions about customer behavior. Additionally, data mining can be used to uncover relationships between different factors that may influence a certain outcome. This type of analysis can be used to create predictive models that can be used to make more accurate predictions about customer behavior.
Conclusion
Data mining applications have become increasingly popular in recent years as businesses and organizations strive to make the most of their data. In order to understand the relationship dynamics of data mining applications, it is important to look at the various types of data mining, the techniques used, and the potential implications of using data mining in various contexts. This article has explored the relationship dynamics of data mining applications and how they can be used to gain insights into customer behavior, market trends, and other valuable data.